Can We Finally Get Past the Fortune-Telling and Teach Students About AI?
AI will keep innovating, and keep surprising
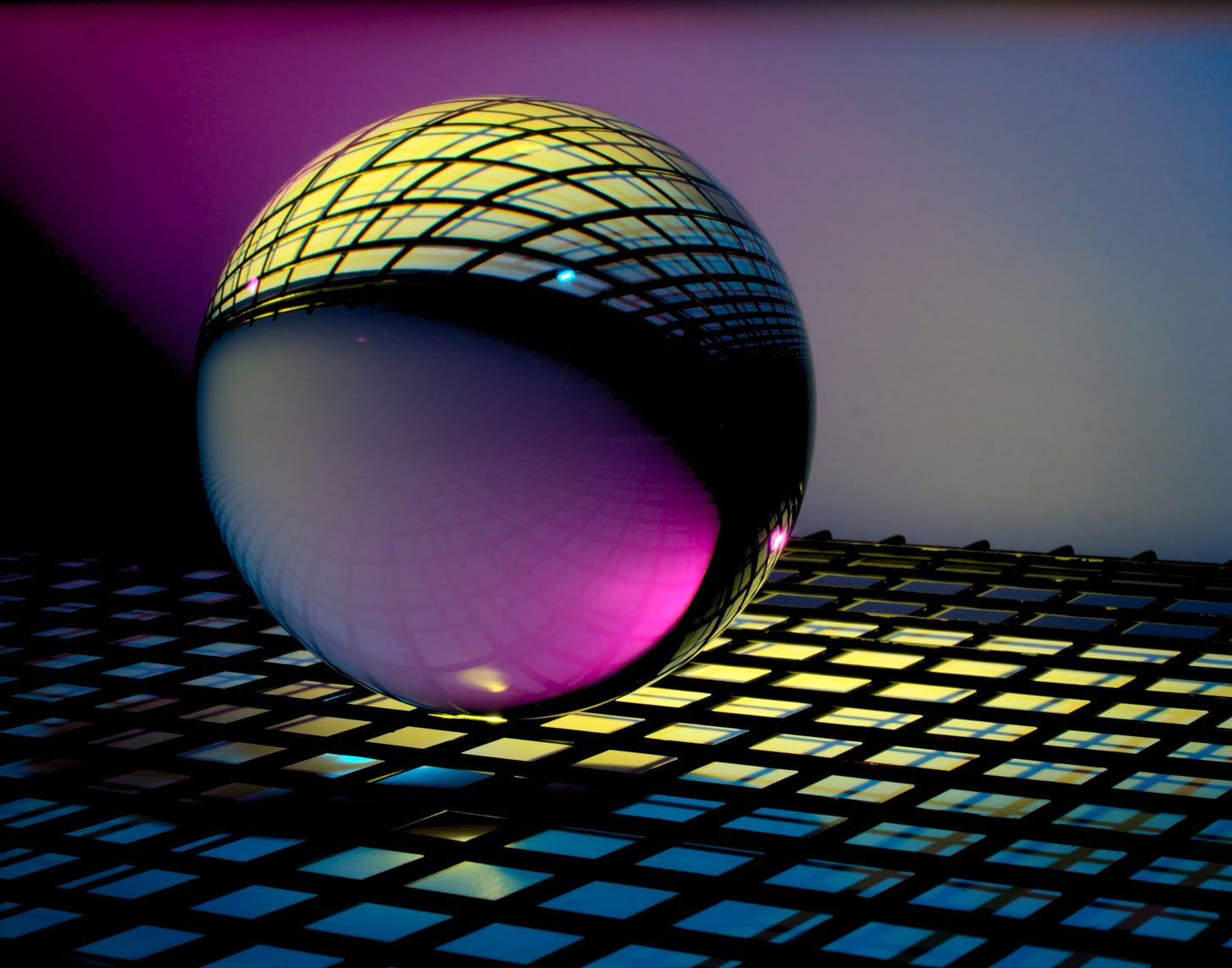
I got a call from someone yesterday who wanted me to talk to investors about the DeepSeek emergence.
DeepSeek is a new Large Language Model (LLM) from Chinese researchers that claims performance that’s in some ways at the level of much bigger LLMs (e.g. ChatGPT, Claude, Gemini) with a much smaller cost to build and use. They claim a $5M development cost, which many are disputing since no evidence or explanation for that claim was presented.
Wall Street is freaking out. The assumption has been that oodles of money and compute are needed to get to the performance levels demonstrated by the leading AIs. Now DeepSeek claims similar capability for a fraction of the cost, and they've open-sourced the methods so the secret sauce isn't secret at all.
I never did talk to the investors, which is wise given I am not a market analyst. It shows the extent of worry that my name even came up!
I have long said the business footprint we see now probably won't be the long-term one. The easiest example is the early Internet, where Netscape faded into oblivion, replaced by better products. But the Internet didn't go away.
The more important realization I hope hits the education community is that human energy is being wasted. Educators conjecture about AI bubbles bursting. They openly express that it's just a fad. They hope for fundamental technical limits (like transformer model limits) to stop progress so we can catch up. They worry about AI sucking up too much electricity and water. But all these hopes rest on the flawed assumption that what we have now with AI is what we will have.
The more important realization that I hope sets into the education community is that all of the energy spent conjecturing and frankly wishing for an AI bubble to burst, showing it's a fad, or for fundamental technical limits to hit and limit the progress so we can catch up, or for AI sucking up way too much energy, are all predicated on one main assumption - that what we have now with AI is what we will have.
We don't account for innovation.
The Innovation Blindspot
In the case of Generative AI (GenAI) such as LLMs, the discounting of innovation has made policy makers, government regulation, and investors look silly. For example, U.S. AI regulation prior to this regime was to pay attention to the size of the AI models, which even at the time I thought was silly since increasingly bigger models wasn’t inevitable. They imposed restrictions on China getting the most advanced AI chips, which evidently just forced more innovation. In AI history, more advanced models were often smaller. This won't be the last time investors overlook innovation.
Schools worry about student assignments being completed by AI, but not about the likely emergent ability for students to send fully functioning avatars to remote class. How will businesses believe the value of a college degree when they can't be sure the student even went to class? Yes, this requires more AI innovation, but I am assuming the innovation will happen based on the progress of the tech.
For decades, Moore's law—really an empirical trend—has shown the power of innovation. Umpteen times researchers have indicated that transistor density, power consumption, and computing power per chip area will stop advancing, that Moore's law will end. It hasn't because of innovation. Tracking the pace of innovation and the ability for a field to overcome prior obstacles is the best indicator for future advance, not whatever methodological constraints currently exist. With AI, the history over the past few decades is that limitations are overcome in less time than AI scientists think. Even they don't account properly for innovation.
For the past two years I've heard Nostradamus predictions that the AI bubble will burst because the models are too expensive to build, that the next advances will take too much money and too much data. "They won't make enough revenue to support the investment," went the logic. The AI bubble might burst. It's happening now to some degree. But not because the models are too expensive to build. It’s the opposite reason. If you think extrapolating current circumstances is a valid way to understand where AI is going, then you're discounting innovation.
Many people want the AI train to slow down, me included. But wishful thinking doesn't make it happen. I even hear many AI scientists not involved directly in developing base Generative AI models expressing those limitations. We latch onto the current limitations and concerns because they are evidenced to some degree by the current situation. Assuming innovation isn't evidence. Rather, it feels unscientific. Yet it's the most reliable aspect of AI's advance.
Why Rapid AI Innovation Will Continue
AI will continue to advance in dramatic ways. At a very abstract level, think of an AI neural network as an ant hill. There are simple units (artificial neurons that do basic math) which when put together and related to one another can do wondrous things, like how ant hills can demonstrate complex behavior from relatively dumb ants. This same phenomenon happens at organizational or societal levels. Putting humans and technology together in large combinations can allow organizations and societies to do (hopefully) wondrous things.
The sophistication of each AI "ant hill" can improve because the basic units are different or the relationships between them are changed. This kind of improvement is shown in the ChatGPT-4 announcement, where parts of the underlying neural net are changed to, for example, have explicit layers that process factual information better.
The neural nets can be scoped differently, as in DeepSeek, where they decided to train lots of smaller, specialist models instead of one big one. This is leaning more on AI teams, the macroscopic innovation direction we've hardly seen applied. Just as individual people can only do so much, big AI models on their own can only do so much.
The AI can debate with themselves, which has been shown to be another direction of improvement somewhat independent from the scaling constraints of the base model. And they can be put together in teams, "organizations," and even societies for additional advancement directions that are likely as big or bigger than the individual model performance advancements. We're not even close to being done with AI advancement, and many feel the advancement will accelerate because of AI helping to build AI. At a minimum, more ideas can be tried.
There are major issues about what to teach about AI, whether and how to teach with AI, and how the answers differ by discipline, student characteristics, and schooling environment. I see far less effort on that topic than I do on environmental impact, AI vs. human, or AI anthropomorphism debates that are much less impactful to students and feel like stalling. They're a distraction from making actual educational progress.
Can we finally get off the hamster wheel where there's lots of consternation but no forward movement? The only sensible way forward is constant, incremental change toward a visionary goal, not thinking about it for years as, well, as schools and colleges have largely been doing.
The pace of change, most of it driven by innovation, is too fast to think you will ever have it all figured out.