(Book Excerpt) Ingredient #1 for Teaching AI Wisdom
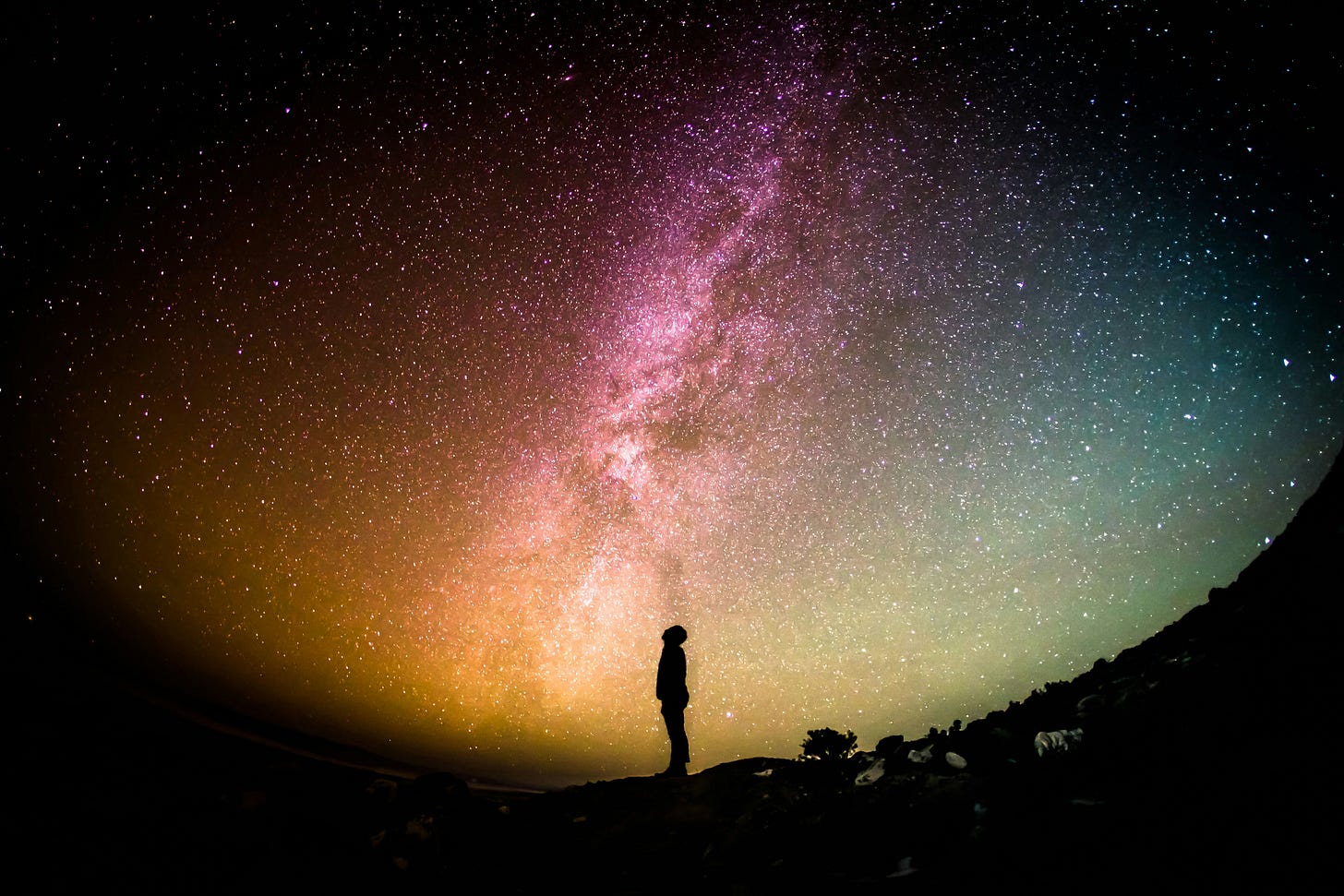
[In my upcoming book—AI Wisdom: Meta-Principles of Thinking and Learning (March 2025)— I describe the durable AI meta-principles that can be taught in any subject. This is an excerpt from Chapter 10 (“Getting Started”), in which I describe the first of five pedagogical conditions for learning those concepts.]
Complex, Open-Ended Challenges
The foundation for learning meta-principles is engaging with challenges that reveal deeper truths. Concepts related to AI can be taught directly. There can be units on how to analyze AI errors or distributed systems, for example. But really understanding these concepts is not only about how to apply the ideas in a specific context but also about what contexts the notion helps. Both of those skills get engaged when the challenge posed is complex and has many different answers.
Let’s say the complex, open-ended challenge is for older students to design an adaptive AI tutoring system for younger students on a particular topic. That’s a topic that pulls in both AI and human teaching considerations.
This isn't a straightforward engineering task. Students must grapple with fundamental questions: How do we know if someone understands something? How do different examples affect learning? When should instruction adapt? What feedback helps versus hinders? These questions naturally lead students to discover key principles about both human and artificial intelligence.
Some students might focus on how to represent knowledge at different levels of understanding. Others could explore how to transform complex ideas into simpler ones. Still others might investigate how to recognize when a learner is confused. Through this process, students encounter multiple meta-principles: how patterns emerge from simple interactions, how concepts get transformed between different representations, and how learning systems need to balance exploration with exploitation.
The challenge's complexity creates natural opportunities to compare human and artificial approaches. Students might realize that both students and AI systems need varied examples to develop robust understanding. They could see how both teachers and an AI used for teaching must transform information based on the learner's current understanding.
These insights directly inform AI interaction. The principles behind effective teaching—context, examples, and information transformation—mirror those in AI prompting. Whether or not students ever use AI for assignments, they're developing deeper intuition about how intelligence processes information.
The open-ended nature prevents students from finding ready-made answers while ensuring discoveries feel genuine. As students progress, they can tackle increasingly sophisticated aspects of the challenge, from basic content adaptation to handling misconceptions to developing more personalized learning paths.
It is very unlikely that such intuitions will arise through narrowly scoped activities and projects. While complexity and ambiguity must be presented appropriately for the student age, the notion that we educate from the bottom-up, building knowledge bricks with the hope that someday the accumulated wall will allow big picture insight, just doesn’t fit what’s needed for abstract conceptual learning. That learning must be instigated from the top-down. In Wisdom Factories I called it upside-down learning. It’s still about learning from what you practice, but to learn abstract concepts you have to practice making decisions on problems that require them.
© 2025 by Dasey Consulting, LLC of Massachusetts. All rights reserved.